Polychronies (2022 / 2025)
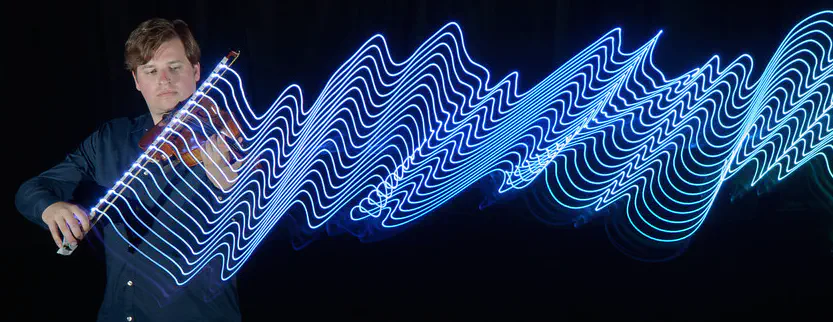
Description
Why do neurons communicate through action potentials, or spikes? An action potential is a binary event —it can occur or not, without further details— and asynchronous, i.e. it can occur at any time. In the living world, neurons almost systematically use this so-called event-based representation, though we do not yet have a clear idea why. A better understanding of this phenomenon remains a fundamental challenge in neurobiology in order to better interpret the masses of recorded data. It is also an emerging challenge in computer science to allow the efficient exploitation of a new class of sensors and impulse computers, called neuromorphic, which could allow significant gains in computing time and energy consumption —a major societal challenge in the age of the digital economy and of global warming.
The goal of this project is to bring an interdisciplinary perspective on the computational advantage of time series representations for the brain and for information processing machines. In particular, we will formalize mathematically a representation in an assembly of neurons based on a set of patterns of different relative spike times called polychronous groups. This hypothesis is directly inspired by neurobiological observations in the hippocampus, and the innovative aspect is to expand the capabilities of analog representations based on the firing rate by considering a representation based on repetitions of these polychronous groups at precise times of occurrence. This formalization is particularly well suited to neuromorphic computing, and allows for supervised or self-supervised learning of polychronous groups in any event-driven data. By extending this paradigm to a hierarchy, we envision practical applications of this approach in audio, video or neurobiological signal processing. The cross-fertilization of neuroscience and neuromimetic approaches will be instrumental in understanding the typical or pathological development of such spiking neural networks.
- grant number AMX-21-RID-025:
- " Ce travail a bénéficié d’une aide du gouvernement français au titre de France 2030, dans le cadre de l’Initiative d’Excellence d’Aix-Marseille Université – A*MIDEX, projet numero AMX-21-RID-025 "
- " This work received support from the french government under the France 2030 investment plan, as part of the Initiative d’Excellence d’Aix-Marseille Université – A*MIDEX, under grant number AMX-21-RID-025 ”
Latest news
- 2023-09-11: Start of post-doc position
- 2023-05-01: Opening of post-doc position (THE POSITION HAS BEEN FILLED!)
- 2022-12-29: check out our review paper: (2022).
- 2022-11-28: Pilot project at the INT brainhack
- 2022-07-18: Le projet Polychronies est lauréat de l’appel à projets « Interdisciplinarité » !
- 2022-02-27: read our complete proposal.