ANR TRAJECTORY (2016/2019)
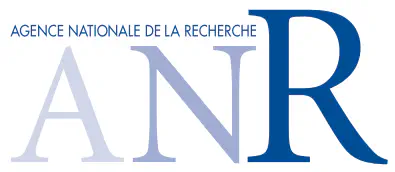
Global motion processing is a major computational task of biological visual systems. When an object moves across the visual field, the sequence of visited positions is strongly correlated in space and time, forming a trajectory. These correlated images generate a sequence of local activation of the feed-forward stream. Local properties such as position, direction and orientation can be extracted at each time step by a feed-forward cascade of linear filters and static non-linearities. However such local, piecewise, analysis ignores the recent history of motion and faces several difficulties, such as systematic delays, ambiguous information processing (e.g., aperture and correspondence problems61) high sensitivity to noise and segmentation problems when several objects are present. Indeed, two main aspects of visual processing have been largely ignored by the dominant, classical feed-forward scheme. First, natural inputs are often ambiguous, dynamic and non-stationary as, e.g., objects moving along complex trajectories. To process them, the visual system must segment them from the scene, estimate their position and direction over time and predict their future location and velocity. Second, each of these processing steps, from the retina to the highest cortical areas, is implemented by an intricate interplay of feed-forward, feedback and horizontal interactions1. Thus, at each stage, a moving object will not only be processed locally, but also generate a lateral propagation of information. Despite decades of motion processing research, it is still unclear how the early visual system processes motion trajectories. We, among others, have proposed that anisotropic diffusion of motion information in retinotopic maps can contribute resolving many of these difficulties25 13. Under this perspective, motion integration, anticipation and prediction would be jointly achieved through the interactions between feed-forward, lateral and feedback propagations within a common spatial reference frame, the retinotopic maps.
Addressing this question is particularly challenging, as it requires to probe these sequences of events at multiple scales (from individual cells to large networks) and multiple stages (retina, primary visual cortex (V1)). “TRAJECTORY” proposes such an integrated approach. Using state-of-the-art micro- and mesoscopic recording techniques combined with modeling approaches, we aim at dissecting, for the first time, the population responses at two key stages of visual motion encoding: the retina and V1. Preliminary experiments and previous computational studies demonstrate the feasibility of our work. We plan three coordinated physiology and modeling work-packages aimed to explore two crucial early visual stages in order to answer the following questions: How is a translating bar represented and encoded within a hierarchy of visual networks and for which condition does it elicit anticipatory responses? How is visual processing shaped by the recent history of motion along a more or less predictable trajectory? How much processing happens in V1 as opposed to simply reflecting transformations occurring already in the retina?
The project is timely because partners master new tools such as multi-electrode arrays and voltage-sensitive dye imaging for investigating the dynamics of neuronal populations covering a large segment of the motion trajectory, both in retina and V1. Second, it is strategic: motion trajectories are a fundamental aspect of visual processing that is also a technological obstacle in computer vision and neuroprostheses design. Third, this project is unique by proposing to jointly investigate retinal and V1 levels within a single experimental and theoretical framework. Lastly, it is mature being grounded on (i) preliminary data paving the way of the three different aims and (ii) a history of strong interactions between the different groups that have decided to join their efforts.
The Marseille team
Frederic Chavane (DR, CNRS, NEOPTO team) is working in the field of vision research for about 20 years with a special interest in the role of lateral interactions in the integration of sensory input in the primary visual cortex. His recent work suggest that lateral interactions mediated by horizontal intracortical connectivity participates actively in the input normalization that controls a wide range of function, from the contrast-response gain to the representation of illusory or real motion. His expertise range from microscopic (intracellular recordings) to mesoscopic (optical imaging, multi-electrode array) recording scales in the primary visual cortex of anesthetized and awake behaving animals.
Laurent Perrinet (CR, CNRS, NEOPTO team). His scientific interests focus on bridging computational understanding of neural dynamics and low-level sensory processing by focusing on motion perception. He is the author of papers in machine learning, computational neuroscience and behavioral psychology. One key concept is the use of statistical regularities from natural scenes as a main drive to integrate local neural information into a global understanding of the scene. In a recent paper that he coauthored (in Nature Neuroscience), he developed a method to use synthesized stimuli targeted to analyze physiological data in a system-identification approach.
Ivo Vanzetta (CR, CNRS, NEOPTO team). His scientific interests focus on how to optimally use photonics-based imaging methods to investigate visual information processing in low-level visual areas, in the anesthetized and awake animal (rodent & primate). As can be seen from his bibliographic record, these methods include optical imaging of intrinsic signals and voltage sensitive dyes and, recently, 2 photon microscopy. Finally I. Vanzetta has an ongoing collaboration with L. Perrinet on the utilization of well-controlled, synthesized nature-like visual stimuli to probe the response characteristics of the primate’s visual system (Sanz-Leon & al. 2012).
Progress meeting ANR TRAJECTORY
- Time January 15th, 2018
- Location INT
- General presentation of the grant, see Anr TRAJECTORY
- Overview of my current projects https://laurentperrinet.github.io/sciblog/files/2017-11-15_ColloqueMaster.html
- MotionClouds with trajectories https://laurentperrinet.github.io/sciblog/posts/2018-01-16-testing-more-complex-trajectories.html or https://laurentperrinet.github.io/sciblog/posts/2018-11-13-testing-more-complex-trajectories.html
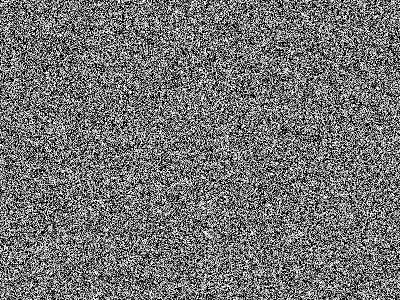
Acknowledgement
This work was supported by ANR project “TRAJECTORY” N° ANR-15-CE37-0011.