ANR Horizontal-V1 (2017/2021)
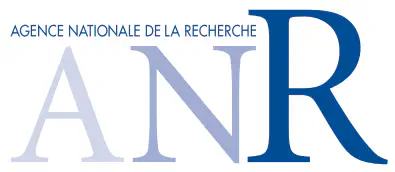
- Description on the official website of the ANR
The Horizontal-V1 project aimed at understanding the emergence of sensory predictions linking local shape attributes (orientation, contour) to global indices of movement (direction, speed, trajectory) at the earliest stage of cortical processing (primary visual cortex, i.e. V1). We studied how the long-distance “horizontal” connectivity, intrinsic to V1 and the feedback from higher cortical areas contribute to a dynamic processing of local-to-global features as a function of the context (eg displacement along a trajectory; during reafference change induced by eye-movements…). We characterized the dynamic processes based on lateral propagation intra-V1, through which spatio-temporal inferences (continuous movement or apparent motion sequences) facilitating spatial (“filling-in”) or positional (“flash-lag”) future expected responses may be generated.
Our main contributions to the project:
- (2021).
- Effect of top-down connections in Hierarchical Sparse Coding. Neural Computation.(2020).
- Pooling in a predictive model of V1 explains functional and structural diversity across species. PLoS Computational Biology.(2022).
- Simulating anticipatory activity in a 1D Spiking Neural Network Model. Bernstein Conference 2021.(2021).
- (2021).
WP3 - Design of novel visual paradigms, probabilistic model of V1 and data-driven simulations - co lead UNIC-INT.
Objectives : This WP will have two primary goals. The first one is theoretically driven, and for sake of simplicity will ignore the dynamic features of neural integration (as expected from a statistical model of image analysis). Binding the different features of visual objects at the local scale (contours) as well as a more global level involves understanding the statistical regularities of the sensory inflow. In particular, titrating the predictions that can be done at the statistical level can be seen as a first pass to better search for critical parameters constraining the network behaviour. From these, we will build probabilistic predictive models optimized for edge co-occurrence classification and generate novel visual statistics 1) which obey rules imposed by the functional horizontal connectivity anisotropies, such as co- circularity, and 2) which facilitate binding in the orientation domain, such as log-polar planforms. These statistics generated in the first half of the grant will be implemented and tested experimentally in the second half of the grant. The second one is more data-driven (as well as phenomenological for feedback from higher cortical areas, since it will not be explored in the grant). Since model fitting will depend on close interactions with WP1 and WP2 measurements, it will be done in the second half of the grant.
WP3-Task 1: Theoretically oriented workplan – Lead INT (Laurent Perrinet)
WP3-Task 1.1 - theory : we will exploit our current expertise in integrating these statistics in the form of probabilistic models to make predictions both at the physiological and modelling levels. First, we will take advantage of our previous work on the quantification of the association field in different classes of natural images (Perrinet & Bednar, 2015). Using an existing library (https://github.com/bicv/SparseEdges), we will use the sparse representation of static natural images to compute histograms of edge co-occurrences. Using an existing algorithm for unsupervised learning (https://github.com/bicv/SparseHebbianLearning), we will learn the different independent components of edge co-occurrences. Such an algorithm fits well a traditional deep-learning convolutional neural network, but, in addition, will include constraints imposed by intra-layer horizontal connectivity. We expect that relevant features will be co-linear or co-circular pairs of edges, but also T-junctions or end-stopping features.
WP3-Task 1.2 - image/film synthesis : We have previously found that random synthetic textures, coined “Motion Clouds”, can be used to quantify V1 implication in visual motion perception (Leon et al, 2012; Simoncini et al, 2012). Recently, the INT and UNIC, partners proved mathematically that these stimuli were optimal with respect to some common geometrical transformations, such as translation, zoom or rotations (Vacher et al, 2015). A main characteristic of these textures is to be generated with a maximally entropic arrangement of elementary textures (so-called textons).
** Informed by the generative model of edge co-occurrences studied in subtask 1, we will be able to extend the family of motion cloud stimuli (Leon et al, 2012; Simoncini et al, 2012) to include joint dependencies between different elements in position or orientation. An exact solution to this problem is hard to achieve as it involves a combinatorial search of all possible combinations of pairs of edges. However, numerous variational approaches are possible and fit well with our probabilistic framework. We will use the convolutional neural network described above but using a back-propagating stream to generate different images. Such a representation will then be optimized using an unsupervised learning method. This is similar to the process used in Generative Adversarial Networks in deep-learning architectures (Radford et al, Archives). ** Finally, the regularities observed in static images will be extended to dynamical scenes by observing that a co-occurrence can be implemented by simple geometrical operations as they are operated in time. For instance a co-circularity is easily described as the set of smooth roto-translational transformations of an edge in time using the group of Galilean transformations (Sarti and Citti, 2006). This theory calls for a first prediction to understand the set of whole possible spatio-temporal co-occurrences of edges as geodesics in the lifted space of all possible trajectories. We predict that such decomposition should allow us to better understand the different classes of features that emerged in the first task.
- WP3-Task 1.3 - Feedback of theory on experimentation : An essential aspect of this work would be to apply these stimuli in neurophysiological experiments and in the modelling. In particular, the ability to select different types of dependencies from the different classes learned above (for instance, co-circularities of a certain curvature range) will make it possible to evaluate the relative contribution of different components of the contextual information. This justifies the fact that the WP3 post-doctoral fellow should have the mobility (between INT and UNIC) and multi-disciplinar profile (theoretical and experimental) to perform this task.
- WP3-Task 1.4 - Generic modelling : These various subtasks will allow us to determine the hierarchy of critical features relevant to describe the full statistics of the space of spatio-temporal edge co-occurrences. Indeed, in static images, we will be able to find independent component in the histograms of edge co-occurrences between metric aspect (distance or scale between edge) from configurational aspects (difference of angle or co-circularity angle).
Similarly, we expect to see that the different independent features should decompose at various scales both in space and in time. For instance, we expect configurational aspects to be more local while aspects related to a motion (Perrinet and Masson, 2012; Khoei et al, 2016) or global shape (form) should be more global. This translates into a probabilistic hierarchical model that would combine dependencies from different cues. In particular, through the emergence of differential pathways for form and motion. These quantitative predictions should finally be confronted at the modelling and neurophysiological levels.
WP3-Task 2 : Data-driven comprehensive model of V1 – Co-lead UNIC and INT
The second task is more data-driven (as well as phenomenological for the feedback circuit part, since largely unknown). Since simulations will depend on close interactions with WP1 and WP2 measurements, it will be developed by the WP3-Post-Doc in the second half of the grant. It will benefit from existing structuro-functional models addressing separately two distinct levels of neural integration, microscopic (conductance-based in Kremkow et al, 2016; Antolik et al, submitted, Chariker et al, 2016) and mesoscopic (VSD-like mean field in Rankin and Chavane, 2017). Efforts will be made to merge these models to fit - in a unified multiscale biologically realistic model - the cellular and VSD data targeting critically horizontal propagation. The parameterization should be flexible enough to produce a generic cortical architecture accounting possibly for species-specificity (Antolik for cat; Chaliker for monkey)
Acknowledgement
This work was supported by ANR project “Horizontal-V1” N° ANR-17-CE37-0006.